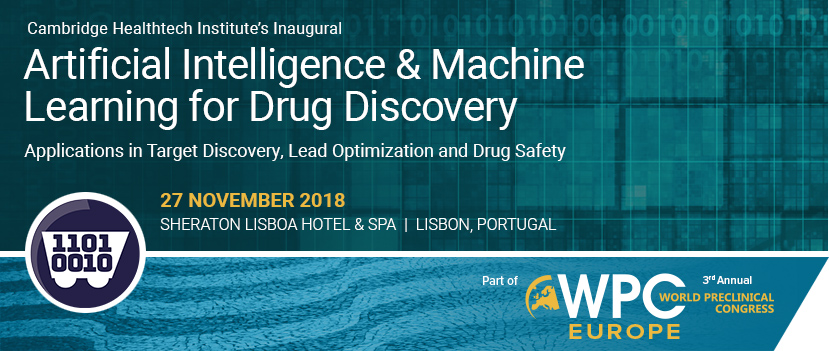
Artificial Intelligence (AI) and Machine Learning (ML) are more than just buzz words being used in the pharmaceutical and biotechnology industry. There is now a steady stream of publications and evidence outlining what these terms really mean, how they
can be applied in a drug discovery and development setting, and how much value they add in terms of saving time, effort and costs. Cambridge Healthtech Institute’s Inaugural AI & Machine Learning for Drug Discovery symposium will bring together computational and bioinformatics experts along with discovery scientists to discuss how some of these technologies and platforms are being used and how well they are living up to their promise. It will include a diverse
set of talks that will highlight how AI and ML can be used for target identification, drug design and optimization, predicting drug toxicity and adverse events. This unique one-day symposium will help attendees meet and interact with experts and peers
from around the world to share ideas and gain some understanding about the opportunities and limitations in using these emerging informatics tools and platforms.
Recommended All Access Package:
27 November: Artificial Intelligence and Machine Learning for Drug Discovery
27 November Dinner Course: SC2: Understanding Key Concepts in Drug Metabolism and Drug Transport
28-29 November: Target Identification and Validation Strategies
29-30 November: CNS Models and Translational Strategies
29 November Dinner Course: SC5: Humanized Mouse Models: Technology and Applications in Preclinical Assessment of Cancer Immunotherapy
Tuesday 27 November
7:30 Registration and Morning Coffee
8:20 Welcome Remarks
Tanuja Koppal, PhD, Conference Director, Cambridge Healthtech Institute
8:25 Chairperson’s Opening Remarks
Maria A. Miteva, PhD, Research Director, Molécules Thérapeutiques in silico (MTi), Inserm Institute
8:30 Introduction to Artificial Intelligence and Machine Learning
Arvind Rao, PhD, Associate
Professor, Department of Computational Medicine and Bioinformatics, University of Michigan
In this introductory talk, we aim to familiarize the audience with basic ideas in machine learning for drug discovery, visiting concepts like dimension reduction, unsupervised and supervised learning using case-studies form drug-target prediction. The
emphasis will be on developing and intuitive understanding of approaches and not on mathematical rigor.
9:00 Overview of How in silico Models Are Being Used to Predict Drug Toxicity
Alexander Amberg, PhD, Computational Toxicologist,
R&D Preclinical Safety, Sanofi
In silico models are used for many different endpoints and in all phases of the drug development process, applied for the safety assessments of drug candidates and targets in the early research until later development
phases, for registration as well as for marketed products. For this many different modelling approaches are used, like statistical structural fragment/fingerprint-based models, molecular descriptor-based QSAR models, systems toxicology models, simulation
models up to newer machine learning models.
9:30 Integrated Mechanistic and Machine Learning Approach for Drug-Drug Interactions
Maria A. Miteva, PhD, Research Director, Molécules Thérapeutiques
in silico (MTi), Inserm Institute
Predicting drug-drug interactions related to inhibition of drug metabolizing enzymes is key for drug toxicity. We will present in silico protocols integrating mechanistic and machine learning approaches to predict the
inhibition of metabolizing enzymes by drug-like molecules and natural compounds. We will focus on cytochrome P450 (CYP), a supergene family of drug metabolizing enzymes responsible for the metabolism of approximately 90% of human drugs and the conjugate
drug metabolizing enzymes sulfotransferases.
10:00 Coffee Break
10:30 Use of a Quantitative Systems Pharmacology (QSP) Model to Predict Liver Toxicity in Simulated Populations
Christina Battista, PhD,
Postdoctoral Fellow, University of North Carolina Institute for Drug Safety Sciences
DILIsym®, the result of an ongoing public-private partnership, has been developed to investigate drug-induced liver injury (DILI). The model employs mathematical representations of mechanistic interactions following drug administration through the
release of serum biomarkers to accurately predict clinical observations in simulated patient populations. This talk will outline DILIsym and discuss applications to date.

11:00 Present and Future Collaborative Drug Discovery Informatics Innovations
Mariana Vaschetto, PhD, Head, Operations, EMEA, Collaborative Drug Discovery
We will present BioAssay Express (BAE) technology, the latest development from CDD. BAE streamlines the conversion of human-readable assay descriptions to computer-readable protocols. We will show how BAE is incorporated in CDD Vault, a secure hosted
platform for storing, mining and sharing chemistry and biology data across multiple organizations.
11:30 Enjoy Lunch on Your Own
13:25 Chairperson’s Remarks
Nicholas P. Tatonetti, PhD, Herbert Irving Assistant Professor of Biomedical Informatics and Director of Clinical Informatics, Herbert Irving Comprehensive Cancer Center, Columbia University
13:30 Drug Discovery at the Speed of Sound
Shabnam Shaabani, PhD, Post-Doctoral Fellow, Drug Design Group, University of Groningen,
The Netherlands
We have introduced a novel approach towards preclinical drug discovery by blending Instant Chemistry, nL dispensing, acoustic-MS, uHTS and artificial intelligence. A prototype instrumentation platform is developed which allows for the parallel synthesis
of hundreds of libraries of scaffolds on an unprecedented dense format. The platform is integrated with acoustic-MS and UPLC-MS for quality control and with different biophysical and phenotypical screening platforms. Artificial intelligence is
developed to ensure never-seen-before fast cycle times for hit-to-lead progression optimizing against multiple parameters at the same time.
14:00 Network-Driven Drug Discovery (NDD) Approach in the Identification and Optimization of Small Molecules
Sree Vadlamudi, PhD, Programme Manager, e Therapeutics plc
We have successfully implemented and validated a highly productive Network-driven Drug Discovery (NDD) platform comprises a suite of powerful, custom computational tools that tap into large-scale proprietary databases, and employs data mining, machine
learning, artificial intelligence, and network science to tackle complex diseases in an efficient and effective way. We will describe a case study highlighting the application of our proprietary NDD methodology in the discovery and optimisation
of small molecules with a novel mechanism of action (MOA).
14:30 KEYNOTE PRESENTATION: Observational Data for Biomedical Discovery
Nicholas P. Tatonetti, PhD, Herbert Irving Assistant
Professor of Biomedical Informatics and Director of Clinical Informatics, Herbert Irving Comprehensive Cancer Center, Columbia University
Data is transforming the scientific method across many domains. In drug safety, data from electronic health records and search logs are now being collected alongside traditional sources. These data sources present new opportunities for studying
the phenomenological and molecular effects of active small molecules. However, they also present new challenges in data integration, statistical analysis, and nature of hypothesis generation. I will discuss these opportunities, challenges
and their role in the future of drug safety science.
15:00 Refreshment Break
15:30 Computational Drug Repurposing Combining Image-Based Screens and Drug-Target Databases
Arvind Rao, PhD, Associate Professor, Department of Computational Medicine and Bioinformatics, University of Michigan
With drug-repurposing being a critical enterprise in mainstream clinical oncology, there is a need for infrastructure to integrate multiple modalities of data to prioritize drug candidates rationally. In this vein, we examine a scenario using
machine learning methods to prioritize genetic modulators selected based on phenotypic (image-based) screening via RNAi. These screen-derived “hits” are then coupled with known drug-target interactions to repurpose candidate drugs
for potential activity in breast cancer cells.
16:00 Computer-Aided Detection and Measurement of Subcutaneous Tumours in Mice
Jamshid Dehmeshki, PhD, Professor of Medical Image Computing and Image Analysis, Department of Computer Science, Kingston University
Andrew Smith, BioVolume Head of Global Commercialisation, Fuel 3D Technologies Limited
This talk will explore how machine learning can aid diagnosis and drug development in cancer research. It will focus on how a novel new 3D measurement solution and machine learning are being used to measure and analyse subcutaneous mice tumours
in major laboratories in the EU and USA to bring about improvements in scientific accuracy and animal welfare while offering the potential to decrease cost and duration of drug development.
16:30 Close of Symposium
18:00 – 20:30 Recommended Dinner Short Course*
SC2: Understanding Key Concepts in Drug Metabolism and Drug Transport
* Separate registration required.